A novel pipeline for drug discovery
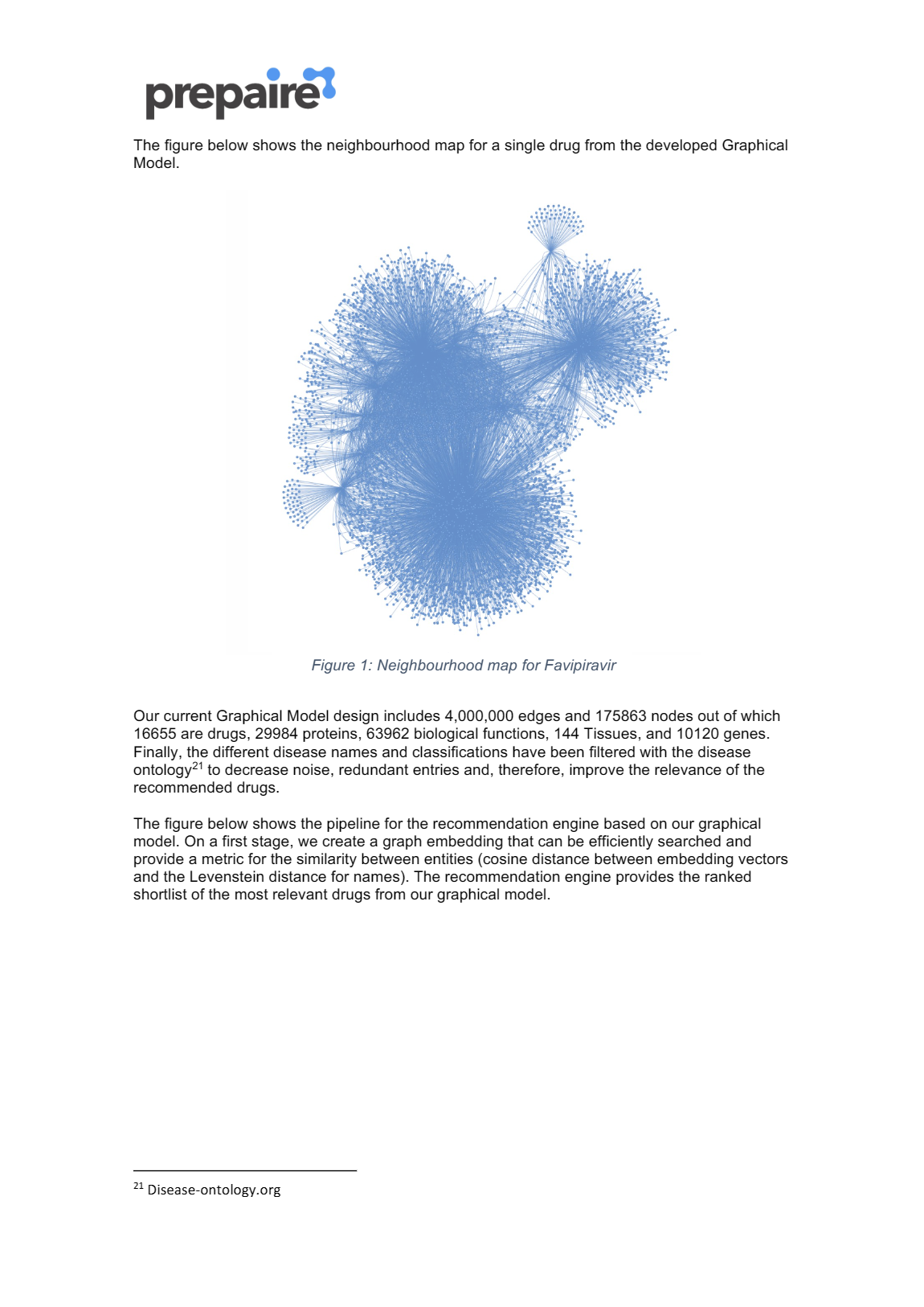
Driven by rapid advances in computer hardware and publicly available datasets over the past decade, deep learning has achieved tremendous success in the transformation of many computational disciplines. These novel technologies have had a considerable impact on computer-aided drug design as well, throughout all stages of the development pipeline.
A flexible toolbox of neural architectures has been developed that is well-suited to represent the sequential, topological, or geometrical concepts of chemistry and biology; and that can either discriminate existing molecules or generate new ones from scratch. Prepaire is using a proprietary algorithm based on Convolutional Deep Neural Networks (CNN)and Generative Adversarial Networks (GANs) to build reactive chemical and biological fitting models enabling the identification ligands to protein targets, protein-protein interactions, generating molecular structures with specified properties combining both functionality and drugability, as well as preparing synthetic data for specific drug discovery and personalized treatment.
Convergence of CRISPR, IPS and Genome sequencing has established a new clinical utility for disease treatment and prevention. Prepaire is enabling precision medicine with intending to integrate whole-genome sequencing with deep phenotyping to data-visualize clinical IPS panels. The platform combines the in-silico prediction with high throughput wet-lab validation in an iterative cycle that empowers continuous improvement and increases efficiency, accuracy, and reliability which are critical to drug R&D. Concurrence of state-of-the art Artificial Intelligence and chemical retrosynthesis has enabled companies like Prepaire to systematically integrate target identification, validation, lead discovery, optimization, drug synthesis, and preclinical testing into a single platform. AI accelerated drug discovery, allowing for a fast-track discovery and repurposing of the existing molecule, intelligent clinical design, and coupled with in-house manufacturing.
Many of the bottlenecks in drug discovery and development could be alleviated if only we could predict earlier in the disease process which drugs are likely to work and for which patients. These platforms accelerate the drug development process by integrating disease models spanning in-vitro cellular systems, and in-silico machine learning models. These models, combined with robotic chemical systems capable of navigating a chemical space based on learned general associations between molecular structures and reactivity, it is possible to identify and predict a range of high yield and highly efficient chemical reactions and products that ultimately lead to the discovery of new molecules or new uses for already existing molecules that can eventually become new speciality treatments. We use artificial intelligence with DL in all accessible formats, including neural, GANs, NLP, supervised, semi-supervised and unsupervised learning to build procedures and pipelines that will become key enablers for medical compliance and clinical approval whilst ensuring IP protection throughout the whole drug discovery process. The successful outcome of these efforts will accelerate all steps of drug discovery and development, including target discovery, lead optimization, toxicity assessment, and trial design.
Read the full article at: (17) (PDF) A novel pipeline for drug discovery (researchgate.net)